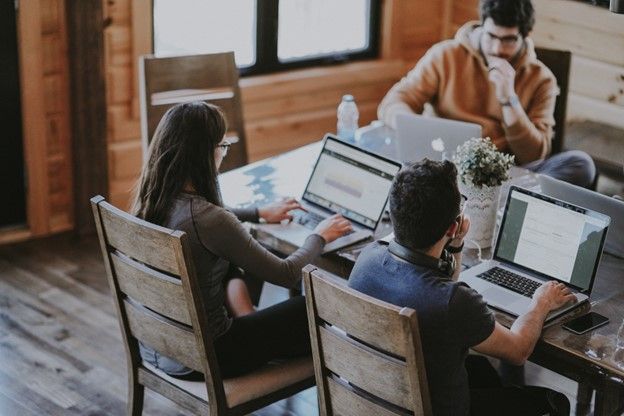
Data analytics and machine learning are increasingly being used in the commercial property sector to optimise property management, leasing, and investment decisions. By using data-driven insights, commercial property professionals can make more informed and strategic decisions.
One way that data analytics and machine learning can be used is to improve property management.
By collecting real-time data from sensors, IoT devices, and other sources which monitor building performance it is possible to identify areas where efficiency can be improved and improve tenant satisfaction:
● Predictive maintenance. It becomes possible to predict when equipment or facilities will need maintenance, and then schedule maintenance proactively, rather than reacting to breakdowns. This can help prevent costly emergency repairs and downtime.
● Data analysis can help property managers identify trends and patterns in equipment performance, which can be used to inform condition-based maintenance schedules. This is a maintenance plan that looks at the condition of a resource to decide what maintenance needs to be done when indicators suggest a decrease in performance. For example if a piece of warehouse machinery begins to overheat.
● Energy management: By looking at energy usage data, property managers can identify areas of high energy consumption and take steps to reduce usage. This can include replacing outdated equipment, improving insulation, or adjusting temperature settings. Lower energy usage means lower costs.
● Asset management: Data analysis can provide property managers with the ability to track all assets, including equipment, facilities and systems.
Another application of data analytics and machine learning in commercial property is in lease optimization. By analysing market trends and tenant data, commercial property owners can determine the optimal lease terms and current rental rates for their properties. Even an ideal tenant for the type of property a landlord owns can be determined and then chosen as to who best matches the criteria.
Once an occupier is in place, machine learning can then monitor tenant behaviour and preferences. This can be collected from several sources including: surveys, social media, sensors in the building and demographic data. The combined data helps landlords identify the types of amenities and services that will retain their chosen tenants.
Finally Data analytics and machine learning can also be used with property investment decisions. Historical transaction data and market trends can help identify investment opportunities and evaluate the potential risks and returns of a particular type of property. Machine learning algorithms can analyse financial and market data in real-time, allowing investors to make more informed and timely investment decisions.
As good as all this sounds there are of course limitations and challenges with using AI in this way. These include:
● The potential for data bias. Data bias can occur when collected information and data comes from non-representative samples or variables are not accounted for or the analyst of the data has an unintentional bias.
● So of course commercial property professionals and businesses need to have the digital skills and knowledge to effectively interpret and act on the insights provided by these technologies.
● Finally the data input needs to be of high-quality. It should be: accurate, complete, reliable, relevant, and timely.
By leveraging real-time data and insights, those involved in commercial property can make more informed and strategic decisions, resulting in improved efficiency, tenant satisfaction, and investment returns. However, it is important to consider the limitations and challenges associated with these technologies and to invest in the skills and knowledge required to effectively use them in the industry